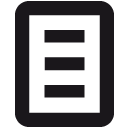
DC Element | Wert | Sprache |
---|---|---|
dc.contributor.advisor | Luxburg, Ulrike von (Prof. Dr.) | |
dc.contributor.author | Kurras, Sven | |
dc.date.accessioned | 2020-10-19T13:18:02Z | - |
dc.date.available | 2020-10-19T13:18:02Z | - |
dc.date.issued | 2016 | |
dc.identifier.uri | https://ediss.sub.uni-hamburg.de/handle/ediss/7263 | - |
dc.description.abstract | Graphs are everywhere. For example, people spend a lot of time on traversing the hyperlink-edges of the web graph. Other examples of graphs are social networks, public transportation maps, molecules, financial transactions, fishing nets, family trees, and the graph that you get by connecting any two natural numbers that have the same digit sum. A graph can be represented by its adjacency matrix W, but there exist several alternative graph matrices. Many structural properties of a graph such as the absence of cycles, number of spanning trees or random walk hitting times, reflect in these graph matrices as all kinds of algebraic properties. This fundamental relation allows to study graph properties by applying all the machinery from linear algebra to matrices. Spectral graph theory focuses particularly on the eigenvalues and eigenvectors of graph matrices. In this field of research, the graph Laplacian matrix L = D − W is particularly well-known, but there are numerous variants such as the normalized Laplacian, the signless Laplacian and the Diplacian. Most variants are defined by a "syntactically tiny" modification of L, for example D + W instead of D − W. Nevertheless, such modifications can drastically affect the information that is encoded in the eigenvalues and eigenvectors. This can finally lead to new relations to graph properties. This thesis studies novel and existing variants of Laplacian matrices. The f-adjusted Laplacian is introduced and proven to be a specific diagonal modification of the normalized Laplacian matrix. In the context of random geometric neighborhood graphs, this matrix can be understood as a specific distortion that is applied to the underlying probability density. This intuition allows for new approaches to various applications in machine learning, for example to image segmentation in case of non-uniformly sampled pixel positions. This thesis further studies the repeated application of the normalization step that underlies the normalized Laplacian. It contributes a novel convergence result that finally leads to the f-fitted Laplacian as another strategy to remove a certain type of sampling bias. The last chapter studies the signed Laplacian, which generalizes the Laplacian to graphs of both positive and negative edge weights. This thesis contributes a novel re-interpretation of the smallest eigenvalue of the signed Laplacian. It identifies the corresponding eigenvector as the canonical candidate for a spectral approach to correlation clustering. The suggested algorithm is shown to compete with state-of-the-art. The algorithm is implemented in an extensive application from practice that aims at automatically detecting unfair user behavior in a multi-player online game. | en |
dc.description.abstract | In sämtlichen Lebensbereichen finden sich Graphen. Zum Beispiel verbringen Menschen viel Zeit mit der Kantentraversierung des Internet-Graphen. Weitere Beispiele für Graphen sind soziale Netzwerke, öffentlicher Nahverkehr, Moleküle, Finanztransaktionen, Fischernetze, Familienstammbäume, sowie der Graph, in dem alle Paare natürlicher Zahlen gleicher Quersumme durch eine Kante verbunden sind. Graphen können durch ihre Adjazenzmatrix W repräsentiert werden. Darüber hinaus existiert eine Vielzahl alternativer Graphmatrizen. Viele strukturelle Eigenschaften von Graphen, beispielsweise ihre Kreisfreiheit, Anzahl Spannbäume, oder Random Walk Hitting Times, spiegeln sich auf die ein oder andere Weise in algebraischen Eigenschaften ihrer Graphmatrizen wider. Diese grundlegende Verflechtung erlaubt das Studium von Graphen unter Verwendung sämtlicher Resultate der Linearen Algebra, angewandt auf Graphmatrizen. Spektrale Graphentheorie studiert Graphen insbesondere anhand der Eigenwerte und Eigenvektoren ihrer Graphmatrizen. Dabei ist vor allem die Laplace-Matrix L = D − W von Bedeutung, aber es gibt derer viele Varianten, zum Beispiel die normalisierte Laplacian, die vorzeichenlose Laplacian und die Diplacian. Die meisten Varianten basieren auf einer "syntaktisch kleinen" Änderung von L, etwa D + W anstelle von D − W. Solcherart Modifikationen ändern meist vollständig die in den Eigenwerten und Eigenvektoren codierte Information. Auf diese Weise können sich neuartige Verbindungen zu Grapheigenschaften ergeben. Die vorliegende Doktorarbeit untersucht neue und existierende Varianten von Laplace-Matrizen. Die f-adjusted Laplacian wird eingeführt und gezeigt, dass diese als eine spezielle Diagonalmodifikation der normalisierten Laplace-Matrix aufgefasst werden kann. Im Kontext zufälliger geometrischer Nachbarschaftsgraphen wird bewiesen, dass diese Matrix eine konkrete Manipulation der zugrundeliegenden Wahrscheinlichkeitsdichte beschreibt. Diese Intuition erlaubt neuartige Ansätze für verschiedene Problemstellungen des Maschinellen Lernens, zum Beispiel für die Bildsegmentierung im Falle nicht-uniform gesampelter Pixelpositionen. Diese Arbeit untersucht zudem die iterierte Anwendung des Normalisierungsschrittes, welcher der normalisierten Laplace-Matrix zugrunde liegt. Das Ergebnis sind neue Resultate zum Konvergenzverhalten. Diese führen zur Definition der f-fitted Laplacian, welche sich beispielsweise zur Behebung eines bestimmten Typs von Stichprobenverzerrung eignet. Das letzte Kapitel studiert die signed Laplacian. Dabei handelt es sich um eine Erweiterung der Laplace-Matrix auf Graphen mit sowohl positiven als auch negativen Kantengewichten. Diese Arbeit bietet eine Neuinterpretation des kleinsten Eigenwertes der signed Laplacian, wodurch sich der zugehörige Eigenvektor als der kanonische Kandidat für spektrales Korrelations-Clustering offenbart. Die Ergebnisse des so entwickelten Algorithmus sind State of the Art. Der Algorithmus wird in einer umfassenden Praxisanwendung implementiert, deren Ziel die automatisierte Identifikation von unfairem Verhalten in einem Multiplayer-Online-Spiel ist. | de |
dc.language.iso | en | en |
dc.publisher | Staats- und Universitätsbibliothek Hamburg Carl von Ossietzky | |
dc.rights | http://purl.org/coar/access_right/c_abf2 | |
dc.subject | Spektrale Graphentheorie | de |
dc.subject | Zufallsgraphen | de |
dc.subject | Korrelations-Clustering | de |
dc.subject | Machine Learning | en |
dc.subject | Spectral Graph Theory | en |
dc.subject | Graph Laplacian | en |
dc.subject | Random Graphs | en |
dc.subject | Correlation Clustering | en |
dc.subject.ddc | 004 Informatik | |
dc.title | Variants of the Graph Laplacian with Applications in Machine Learning | en |
dc.title.alternative | Varianten der Graph Laplacian mit Anwendungen im Maschinellen Lernen | de |
dc.type | doctoralThesis | |
dcterms.dateAccepted | 2017-03-22 | |
dc.rights.cc | No license | |
dc.rights.rs | http://rightsstatements.org/vocab/InC/1.0/ | |
dc.subject.bcl | 31.12 Kombinatorik, Graphentheorie | |
dc.subject.bcl | 31.73 Mathematische Statistik | |
dc.subject.bcl | 54.10 Theoretische Informatik | |
dc.subject.gnd | Graphentheorie | |
dc.subject.gnd | Maschinelles Lernen | |
dc.subject.gnd | Statistik | |
dc.subject.gnd | Laplace-Operator | |
dc.subject.gnd | Unüberwachtes Lernen | |
dc.subject.gnd | Kernschätzung | |
dc.type.casrai | Dissertation | - |
dc.type.dini | doctoralThesis | - |
dc.type.driver | doctoralThesis | - |
dc.type.status | info:eu-repo/semantics/publishedVersion | |
dc.type.thesis | doctoralThesis | |
tuhh.opus.id | 8595 | |
tuhh.opus.datecreation | 2017-07-27 | |
tuhh.type.opus | Dissertation | - |
thesis.grantor.department | Informatik | |
thesis.grantor.place | Hamburg | |
thesis.grantor.universityOrInstitution | Universität Hamburg | |
dcterms.DCMIType | Text | - |
tuhh.gvk.ppn | 897286340 | |
dc.identifier.urn | urn:nbn:de:gbv:18-85957 | |
item.creatorOrcid | Kurras, Sven | - |
item.creatorGND | Kurras, Sven | - |
item.languageiso639-1 | other | - |
item.fulltext | With Fulltext | - |
item.advisorGND | Luxburg, Ulrike von (Prof. Dr.) | - |
item.grantfulltext | open | - |
Enthalten in den Sammlungen: | Elektronische Dissertationen und Habilitationen |
Dateien zu dieser Ressource:
Datei | Beschreibung | Prüfsumme | Größe | Format | |
---|---|---|---|---|---|
Dissertation.pdf | 0628c7d687eb53758ccf3e43df4a8907 | 15.61 MB | Adobe PDF | Öffnen/Anzeigen |
Diese Publikation steht in elektronischer Form im Internet bereit und kann gelesen werden. Über den freien Zugang hinaus wurden durch die Urheberin / den Urheber keine weiteren Rechte eingeräumt. Nutzungshandlungen (wie zum Beispiel der Download, das Bearbeiten, das Weiterverbreiten) sind daher nur im Rahmen der gesetzlichen Erlaubnisse des Urheberrechtsgesetzes (UrhG) erlaubt. Dies gilt für die Publikation sowie für ihre einzelnen Bestandteile, soweit nichts Anderes ausgewiesen ist.
Info
Seitenansichten
1.031
Letzte Woche
Letzten Monat
geprüft am 17.06.2025
Download(s)
1.203
Letzte Woche
Letzten Monat
geprüft am 17.06.2025
Werkzeuge