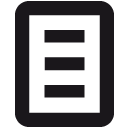
DC Element | Wert | Sprache |
---|---|---|
dc.contributor.advisor | Biemann, Chris (Prof. Dr.) | |
dc.contributor.author | Yimam, Seid Muhie | |
dc.date.accessioned | 2020-10-19T13:24:05Z | - |
dc.date.available | 2020-10-19T13:24:05Z | - |
dc.date.issued | 2019 | |
dc.identifier.uri | https://ediss.sub.uni-hamburg.de/handle/ediss/8301 | - |
dc.description.abstract | Most applications in natural language processing (NLP) are based on machine learning components. Most of these components need to be trained in a supervised way and require a substantial amount of training data. These training data have to be manually labeled or annotated by domain experts. Creating such dataset is a challenging task: first, domain experts 1) need to know what to annotate and how to annotate (compiling an annotation guideline), 2) study if existing annotation tools are adequate or develop a new annotation tool, and 3) assess the quality of annotated data. Second, the annotation task is expensive mainly to compensate the annotators. Third, the annotation task takes much time to collect enough data. Finally, the collected data might get obsolete if the requirement of the target NLP application changes overm time, which is known as concept drift or semantic drift. This work tackles three main questions: how to build rapid annotation tools, how to build and integrate resources to NLP applications, and how to integrate adaptive models into NLP applications. A rapid annotation approach focuses on completing the task much quicker by employing different strategies within the annotation tool. For the development of an adaptive and personalized NLP application, we embed an adaptive machine learning model into the application that can continuously learn and update its model from usage data. The thesis comprises of five parts. Part I describes the problem statements, research problems, and background of the study, Part II presents the annotation and NLP tools we have developed during the thesis work, Part III discusses the approaches used to collect semantic-aware NLP resources, Part IV describes the experimental setups and results, and finally Part V concludes with main findings and results of the thesis. In Part I, we present the main goals of the thesis (see Chapter 1) and discuss the foundations of the rapid annotations and adaptive NLP technologies (see Chapter 2). Part II of the thesis presents the different tools we design for rapid, adaptive, and personalized annotations. This part describes the technological basis, which needs to be especially robust because of the user-interfacing nature of the experiments. In Chapter 3, we discuss the design and implementation approaches for the development of the WebAnno online annotation tool. WebAnno incorporates different rapid annotation properties such as support of annotation correction, automation to produce annotation suggestions, and built-in annotation adjudication. We also extend WebAnno with different user interface components that are meantto facilitate rapid annotation. In Chapter 4, we present Par4Sem, a semantic-aware writing aid tool that enhances document composing with an adaptive and personalized paraphrasing component. The tool mimics a standard word processor, but it has an integrated adaptive model that provides semantic-aware text paraphrasing capability. In Chapter 5, we present the different information visualization (InfoVis) tools, which can be used to collect training data as part of data visualization and exploration. In Part III of the thesis, we discuss data collection, experimental setups, and results obtained particularly for semantic-aware NLP applications. In Chapter 6, we investigate the impact of context for the paraphrasing tasks. Paraphrasing is an approach of re-writing texts to produce equivalent texts that convey the same information. For this experiment, we produce candidate paraphrases for target words or phrases from different paraphrase resources and present the candidates for multiple crowdsourcing workers to re-rank candidates based on their context. The results obtained show that awareness of context improves paraphrase ranking. In Chapter 7, we investigate whether native and non-native language speakers have different demands for text simplification or not. We first conduct complex words or phrases identification (CWI) annotation task, where the goal is to determine parts of a text that could pose difficulty to understand a text. Then, we investigate if machine learning models build for one language, using de-lexicalized features, can be used to predict complex phrases for 1) different user groups (native and non-native users), 2) different text genres (Wikipedia and news articles), and 3) different languages (a CWI model trained for English can be used to identify complex phrases for German). The most important finding is that it is possible to build a CWI system for onem language and use the model to identify complex words or phrases for other languages. Part IV contains the main experimental results for the setups of annotation automation and an adaptive NLP application. In Chapter 8, we conduct and evaluate different rapid and automation annotation experiments using the WebAnno annotation tool. Regarding the annotation time using the WebAnno automation component, we demonstrate that the rapid annotation approach speeds up the annotation process by the factor of 3 to 4 on sequence tagging tasks. In an experiment with biomedical named entity recognition, the adaptive component of WebAnno allows for fast and easy annotation of medical entities where the model suggests useful entities already after the annotation of a handful of medical abstracts. In Chapter 9, we discuss the results and implications of the experiments on adaptive and personalized NLP application. An adaptive and personalized NLP application is realized by integrating adaptive machine learning models into a semantic writing aid tool (Par4Sem). The first adaptive model is a complex word identification component, which adaptively learns the complex or difficult words from the user interaction. We instantiate the adaptive model using a baseline system of the CWI datasets. The second component is a paraphrase ranking model, which learns to rank or order candidate paraphrases adaptively. We experiment using the Amazon Mechanical Turk (MTurk) crowdsourcing where Par4Sem is explicitly used to simplify texts for a given target reader (children, language learners, or people with reading impairment). To test the adaptability of the models, we run the experiments for 9 iterations where the models are updated for each iteration. The experimental results for the paraphrase ranking show that in every iteration, there is a substantial increase in performance based on the normalized discounted cumulative gain (NDCG) learning to rank evaluation metrics. In conclusion, with the rapid advancement of machine learning strategies, the integration of adaptive models into the application with a self-updating capability is a way forward. Moreover, as machine learning models are becoming prevalent in many NLP applications and software systems, a generic model will have limitations in fulfilling the target application’s requirement. Instead of investing even more efforts in collecting training datasets and training a static machine learning model, we should focus on building an adaptive and personalized model that is capable of learning continuously from the user interaction. | en |
dc.description.abstract | Die meisten Anwendungen im Bereich Sprachverarbeitung (Natural Language Processing, NLP) basieren auf Komponenten des Maschinellen Lernens. Die meisten dieser Komponenten müssen in einem überwachten Setting trainiert werden und benötigen hierfür ausreichend Trainingsdaten, die von Domänen-Experten manuell gelabelt oder annotiert werden müssen. Die Erstellung eines solchen Datensatzes bietet viele Herausforderungen: zunächst sollen die Domänen- Experten 1) wissen, was zu annotieren ist und wie man annotiert (Erstellung von Annotations- Guidelines), 2) prüfen, ob die existierenden Annotations-Tools ausreichend sind, oder ein neues Annotations-Tool erstellen, und 3) die Qualität der annotierten Daten überprüfen. Zweitens ist das Annotieren kostenaufwendig, vor allem um die Annotatoren zu bezahlen. Drittens dauert das Annotieren sehr lange, wenn man viele Daten sammeln will. Zuletzt können die gesammelten Daten auch veralten, wenn die Anforderungen an die NLP-Anwendung sich mit der Zeit ändern. Das nennt sich semantische oder Konzept-Verschiebung. Diese Doktorarbeit will drei Ziele erreichen: die Erstellung von schnellen Annotations- Tools, die Erstellung und Integration von Sprachressourcen für NLP-Anwendungen und die Integration von adaptiven Modellen in NLP-Anwendungen. Der Ansatz des schnellen Annotierens (rapid annotation) setzt den Akzent auf die schnelle Erledigung des Annotations- Tasks, indem verschiedene Strategien direkt im Tool implementiert werden. Um eine adaptive und personalisierte NLP-Anwendung zu entwickeln, bauen wir ein adaptives maschinell lernendes Modell in die Anwendung ein, das durch die Benutzung ständig lernen und das Modell aktualisieren kann. Die Doktorarbeit besteht aus fünf Teilen. Teil I beschreibt die Problemstellung an sich, wissenschaftliche Probleme und den Hintergrund dieser Studie, Teil II stellt die Annotationen und die NLP-Tools vor, die wir in der Arbeit an dieser Doktorarbeit entwickelt haben. Teil III erörtert die Ansätze, die für das Sammeln von NLP-Ressourcen mit Semantik-Kenntnissen benutzt werden, und Teil IV beschreibt die Versuchsaufbauten und die Ergebnisse. Zuletzt schließt Teil V mit den wichtigsten Ergebnissen und Erkenntnissen aus dieser Doktorarbeit ab. Im Teil I stellen wir die Hauptziele der Doktorarbeit vor (siehe Kapitel 1) und diskutieren die Grundzüge des schnellen Annotierens und der adaptiven NLP-Technologien (siehe Kapitel 2). Teil II dieser Doktorarbeit stellt die verschiedenen Tools vor, die wir für die schnelle, adaptive und personalisierte Annotation entwickelt haben. Im Kapitel 3 erläutern wir das Design und die Implementierung des online Annotations-Tools WebAnno. WebAnno erfüllt verschiedene Anforderungen und Eigenschaften des schnellen Annotierens, wie die Unterstützung von Annotations-Korrekturen, Automation um Annotationsvorschläge zu generieren und eingebautes Bewerten von Annotationsentscheidungen. Außerdem haben wir WebAnno mit verschiedenen Komponenten in der Benutzeroberfläche ausgestattet, die schnelles Annotieren erleichtern sollen. Im Kapitel 4 stellen wir Par4Sem vor, eine semantische Schreibhilfe, welche die Erstellung von Dokumenten mit einer personalisierten Paraphrasen-Komponente erleichtert. Das Tool ahmt ein Textverarbeitungssystem nach, aber es nutzt ein integriertes adaptives Modell, das lexikalisches Paraphrasieren anbietet. Im Kapitel 5 stellen wir verschiedene Informations- Visualisierungstools (InfoVis) vor, die für das Sammeln von Trainings-Daten benutzt werden können und Teil von Daten-Visualisierungen und Erkundung sind. Im Teil III dieser Doktorarbeit erörtern wir die Datensammlung, Versuchsaufbauten und die Ergebnisse, die wir für semantische NLP-Anwendungen gesammelt haben. Im Kapitel 6 untersuchen wir den Einfluss des Kontextes auf die Paraphrasierung. Paraphrasierung ist ein Ansatz um Texte umzuschreiben und dabei äquivalente Texte mit dem gleichen Informationsgehalt zu generieren. Für dieses Experiment erstellen wir aus verschiedenen Paraphrasen-Ressourcen Paraphrase-Kandidaten für Zielwörter und Phrasen und präsentieren diese Kandidaten mehreren Crowdworkern, damit diese die Kandidaten im gegebenen Kontext nach Reihenfolge ordnen können. Die erzielten Ergebnisse zeigen, dass der Kontext-Bezug das Paraphrasieren verbessert. Im Kapitel 7 untersuchen wir, ob Muttersprachler andere Anforderungen an Textvereinfachung stellen als Nicht-Muttersprachler. Dazu führen wir eine Annotationsaufgabe durch, der komplexe Wörter und Phrasen ermittelt (complex word identification, CWI). Das Ziel dabei ist es, Teile eines Texts auszuzeichnen, die schwierig zu verstehen sein können. Dann prüfen wir, ob Modelle des maschinellen Lernens, die auf einer Sprache trainiert wurden, mittels delexikalisierten Features dazu genutzt werden können, komplexe Phrasen vorauszusagen für 1) verschiedene Nutzergruppen (Muttersprachler und Nicht-Muttersprachler), 2) verschiedene Textgattungen (Wikipedia und Nachrichtentexte) und 3) verschiedene Sprachen (ein auf Englisch trainiertes CWI-Modell wird für die Erkennung komplexer Phrasen im Deutschen verwendet). Die wichtigste Erkenntnis ist, dass es möglich ist, ein CWI-System für eine Sprache zu entwickeln und dessen Modell für die Erkennung von komplexen Wörtern und Phrasen einer anderen Sprache zu nutzen. Im Teil IV präsentieren wir die experimentellen Ergebnisse verschiedener Arten von Annotationsautomation und adaptiver NLP-Anwendungen. Im Kapitel 8 führen wir verschiedene Experimente für schnelles und automatisiertes Annotieren mittels des WebAnno-Tools durch und evaluieren diese. Bezüglich der Annotations-Zeit mit der Automation-Komponente von WebAnno, zeigen wir, dass der Ansatz des schnellen Annotierens den Annotations-Vorgang bei einer sequentiellen Annotationsaufgabe um den Faktor 3 bis 4 beschleunigt. In einem Experiment für das Erkennen von biomedizinischen Entitäten (biomedical Named Entity Recognition), ermöglicht die adaptive Komponente von WebAnno eine schnelle und einfache Annotation von medizinischen Entitäten, wo das Modell bereits mögliche Entitäten vorschlägt, nachdem es anhand von wenigen annotierten medizinischen Abstracts mitgelernt hat. Im Kapitel 9 diskutieren wir die Ergebnisse und Folgerungen der Experimente zu adaptiven und personalisierten NLP-Anwendungen. Eine adaptive und personalisierte NLP-Anwendung wird durch die Integration von adaptiven Modellen des Maschinellen Lernens in einer semantische Schreibhilfe realisiert (Par4Sem). Das erste adaptive Modell ist eine Komponente zur Erkennung von komplexen Wörtern, die adaptiv komplexe oder schwierige Wörter durch Benutzerinteraktion lernt. Wir instanziieren das adaptive Modell durch ein Baseline-System der CWI-Datensätze. Die zweite Komponente ist ein Paraphrasen-Ranking-Modell, das adaptiv lernt, wie man die Paraphrasen-Kandidaten ordnet. Wir führen die Experimente mit der Crowdsourcing Plattform Amazon Mechanical Turk (MTurk) durch, wo Par4Sem explizit dafür verwendet wird, Texte für eine bestimmte Nutzergruppe zu vereinfachen (Kinder, Sprachenlernende oder Menschen mit Lesebeeinträchtigungen). Um die Anpassungsfähigkeit des Modells zu testen, führen wir ein Experiment mit 9 Interationen durch und aktualisieren das Modell nach jeder Iteration. Die Ergebnisse des Paraphrasen-Rankings anhand der Ranking-Metrik NDCG (normalized discounted cumulative gain) zeigen, dass die Performanz sich substantiellverbessert. Abschließend zeigt sich, dass bei der schnellen Fortentwicklung der Strategien des Maschinellen Lernens die Integration adaptiver Modelle in einer Anwendung mit selbst-aktualisierenden Fähigkeiten Vorteile bietet. Zudem wird ein generisches Modell in NLP-Anwendungen und Systemen, die zunehmend auf Maschinelles Lernen setzen, die Erfüllung der Anforderungen an solche Anwendungen beschränken. Anstatt viel Energie darauf zu verwenden, Trainingsdaten zu sammeln und ein Modell zu trainieren und anzupassen, das möglicherweise schnell überholt ist, sollten wir unseren Fokus auf adaptive und personalisierte Modelle setzen, die beständig durch Benutzerinteraktion lernen können. | de |
dc.language.iso | en | en |
dc.publisher | Staats- und Universitätsbibliothek Hamburg Carl von Ossietzky | |
dc.rights | http://purl.org/coar/access_right/c_abf2 | |
dc.subject | Annotation | en |
dc.subject | NLP | en |
dc.subject | adaptive learning | en |
dc.subject | data collection | en |
dc.subject | annotation tool | en |
dc.subject | machine learning | en |
dc.subject | simplification | en |
dc.subject | writing aid | en |
dc.subject | paraphrasing | en |
dc.subject.ddc | 004 Informatik | |
dc.title | Adaptive Approaches to Natural Language Processing in Annotation and Application | en |
dc.title.alternative | Adaptive Ansätze zur Verarbeitung natürlicher Sprache in Annotation und Anwendung | de |
dc.type | doctoralThesis | |
dcterms.dateAccepted | 2019-07-03 | |
dc.rights.cc | No license | |
dc.rights.rs | http://rightsstatements.org/vocab/InC/1.0/ | |
dc.subject.bcl | 54.72 Künstliche Intelligenz | |
dc.subject.bcl | 54.75 Sprachverarbeitung | |
dc.type.casrai | Dissertation | - |
dc.type.dini | doctoralThesis | - |
dc.type.driver | doctoralThesis | - |
dc.type.status | info:eu-repo/semantics/publishedVersion | |
dc.type.thesis | doctoralThesis | |
tuhh.opus.id | 9914 | |
tuhh.opus.datecreation | 2019-08-12 | |
tuhh.type.opus | Dissertation | - |
thesis.grantor.department | Informatik | |
thesis.grantor.place | Hamburg | |
thesis.grantor.universityOrInstitution | Universität Hamburg | |
dcterms.DCMIType | Text | - |
tuhh.gvk.ppn | 1676123083 | |
dc.identifier.urn | urn:nbn:de:gbv:18-99140 | |
item.advisorGND | Biemann, Chris (Prof. Dr.) | - |
item.grantfulltext | open | - |
item.languageiso639-1 | other | - |
item.fulltext | With Fulltext | - |
item.creatorOrcid | Yimam, Seid Muhie | - |
item.creatorGND | Yimam, Seid Muhie | - |
Enthalten in den Sammlungen: | Elektronische Dissertationen und Habilitationen |
Dateien zu dieser Ressource:
Datei | Beschreibung | Prüfsumme | Größe | Format | |
---|---|---|---|---|---|
Dissertation.pdf | 726cabc91deec53246d480750ed9b8ad | 9.44 MB | Adobe PDF | Öffnen/Anzeigen |
Diese Publikation steht in elektronischer Form im Internet bereit und kann gelesen werden. Über den freien Zugang hinaus wurden durch die Urheberin / den Urheber keine weiteren Rechte eingeräumt. Nutzungshandlungen (wie zum Beispiel der Download, das Bearbeiten, das Weiterverbreiten) sind daher nur im Rahmen der gesetzlichen Erlaubnisse des Urheberrechtsgesetzes (UrhG) erlaubt. Dies gilt für die Publikation sowie für ihre einzelnen Bestandteile, soweit nichts Anderes ausgewiesen ist.
Info
Seitenansichten
1.514
Letzte Woche
Letzten Monat
geprüft am 29.06.2024
Download(s)
876
Letzte Woche
Letzten Monat
geprüft am 29.06.2024
Werkzeuge