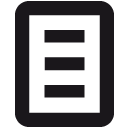
DC Element | Wert | Sprache |
---|---|---|
dc.contributor.advisor | Zhang, Jianwei (Prof. Dr.) | |
dc.contributor.author | Mi, Jinpeng | |
dc.date.accessioned | 2020-10-19T13:28:01Z | - |
dc.date.available | 2020-10-19T13:28:01Z | - |
dc.date.issued | 2020 | |
dc.identifier.uri | https://ediss.sub.uni-hamburg.de/handle/ediss/8361 | - |
dc.description.abstract | Natural language provides an intuitive and effective interaction interface between human beings and intelligent agents. Currently, multiple approaches have been proposed to address natural language visual grounding. However, most of the existing approaches alleviate the ambiguity of natural language queries and achieve target objects grounding by drawing support from auxiliary information, such as dialogues between human users, and gestures. While the auxiliary information-based systems usually make the natural language grounding cumbersome and time-consuming. This thesis aims to study and exploit multimodal learning approaches for natural language visual grounding. Inspired by the pattern of human beings understanding and grounding target objects according to given natural language queries, we propose different architectures to address natural language visual grounding. First, we propose a semantic-aware network for referring expression comprehension which aims to locate the most relevant objects in images given natural referring expressions. The proposed referring expression comprehension network excavates the visual semantics in images via a visual semantic-aware network, exploits the rich linguistic contexts in referring expressions by a language attention network, and locates target objects by integrating the outputs of the visual semantic-aware network and the language attention network. Moreover, we conduct extensive experiments on three public datasets to validate the performance of the presented network. Second, we present a Generative Adversarial Networks-based network to generate diverse and natural referring expressions. Referring expression generation mimics the role of a speaker to generate referring expressions for each detected region within images. For this task, we aim to improve the diversity and naturalness of expressions without sacrificing semantic validity. To this end, we propose a generator to generate expressions and exploit a discriminator to classify whether the generated descriptions are real or fake. We evaluate the performance of the proposed generation network via multiple evaluation metrics. Third, inspired by the psychology term “affordance” and its applications in Human-Robot interaction, we draw support from object affordance to ground intention-related natural language queries. Formally, we first present an attention-based multi-visual features fusion network to recognize object affordances. The proposed network fuses deep visual features extracted from a pretrained CNN model with deep texture features encoded by a deep texture encoding network via an attention-based mechanism. We train and validate the performance of the object affordance detection network on a self-built dataset. Moreover, we propose three natural language visual grounding architectures, which are based on referring expression comprehension, referring expression generation, and object affordance detection, respectively. We combine the referring expression comprehension and referring expression generation models with scene graph parsing to achieve complicated and unconstrained natural language queries grounding. Additionally, we integrate the object affordance detection network with an intention semantic extraction module and a target grounding module to ground intention-related natural language queries. Finally, we implement extensive experiments to validate the effectiveness of the presented natural language visual grounding architectures. We also integrate with an online speech recognizer to complete target object grounding and manipulation experiments on a PR2 robot given spoken natural language commands. | en |
dc.language.iso | en | en |
dc.publisher | Staats- und Universitätsbibliothek Hamburg Carl von Ossietzky | |
dc.rights | http://purl.org/coar/access_right/c_abf2 | |
dc.subject.ddc | 004 Informatik | |
dc.title | Natural Language Visual Grounding via Multimodal Learning | en |
dc.title.alternative | Natürliche Sprache Visual Grounding durch multimodales Lernen | de |
dc.type | doctoralThesis | |
dcterms.dateAccepted | 2020-01-20 | |
dc.rights.cc | No license | |
dc.rights.rs | http://rightsstatements.org/vocab/InC/1.0/ | |
dc.subject.bcl | 54.72 Künstliche Intelligenz | |
dc.type.casrai | Dissertation | - |
dc.type.dini | doctoralThesis | - |
dc.type.driver | doctoralThesis | - |
dc.type.status | info:eu-repo/semantics/publishedVersion | |
dc.type.thesis | doctoralThesis | |
tuhh.opus.id | 10263 | |
tuhh.opus.datecreation | 2020-02-17 | |
tuhh.type.opus | Dissertation | - |
thesis.grantor.department | Informatik | |
thesis.grantor.place | Hamburg | |
thesis.grantor.universityOrInstitution | Universität Hamburg | |
dcterms.DCMIType | Text | - |
tuhh.gvk.ppn | 1691677736 | |
dc.identifier.urn | urn:nbn:de:gbv:18-102632 | |
item.fulltext | With Fulltext | - |
item.creatorOrcid | Mi, Jinpeng | - |
item.advisorGND | Zhang, Jianwei (Prof. Dr.) | - |
item.languageiso639-1 | other | - |
item.creatorGND | Mi, Jinpeng | - |
item.grantfulltext | open | - |
Enthalten in den Sammlungen: | Elektronische Dissertationen und Habilitationen |
Dateien zu dieser Ressource:
Datei | Beschreibung | Prüfsumme | Größe | Format | |
---|---|---|---|---|---|
Dissertation.pdf | 2f82d3b505c248606ebe8df4d9a021e5 | 6.66 MB | Adobe PDF | Öffnen/Anzeigen |
Diese Publikation steht in elektronischer Form im Internet bereit und kann gelesen werden. Über den freien Zugang hinaus wurden durch die Urheberin / den Urheber keine weiteren Rechte eingeräumt. Nutzungshandlungen (wie zum Beispiel der Download, das Bearbeiten, das Weiterverbreiten) sind daher nur im Rahmen der gesetzlichen Erlaubnisse des Urheberrechtsgesetzes (UrhG) erlaubt. Dies gilt für die Publikation sowie für ihre einzelnen Bestandteile, soweit nichts Anderes ausgewiesen ist.
Info
Seitenansichten
622
Letzte Woche
Letzten Monat
geprüft am 06.07.2025
Download(s)
446
Letzte Woche
Letzten Monat
geprüft am 06.07.2025
Werkzeuge