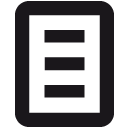
DC Element | Wert | Sprache |
---|---|---|
dc.contributor.advisor | Menzel, Wolfgang | - |
dc.contributor.advisor | Vertan, Cristina | - |
dc.contributor.advisor | von Hahn, Walther | - |
dc.contributor.author | Duma, Mirela-Stefania | - |
dc.date.accessioned | 2022-08-04T11:43:15Z | - |
dc.date.available | 2022-08-04T11:43:15Z | - |
dc.date.issued | 2021 | - |
dc.identifier.uri | https://ediss.sub.uni-hamburg.de/handle/ediss/9721 | - |
dc.description.abstract | Machine Translation (MT) is a current topic in the Computational Linguistics (CL) community. Training an MT model on a domain and using it on another domain does not yield the expected performance due to the syntactic and semantic differences between the two domains. Thus, domain adaptation is necessary. Data selection, which is the topic of this thesis, is a corpus-driven domain adaptation method. Given a general domain corpus and an in-domain, each sentence from the general domain corpus is scored according to its similarity to the in-domain. The most similar sentences to an in-domain are selected as pseudo in-domain and used later on in the training of domain-focused MT systems. There are two challenges that arise with data selection: which method to use to determine the most similar sentences from the general domain to a given in-domain and how many of the general domain sentences to select as pseudo in-domain. In this thesis, data selection methods that address both challenges are presented. I developed several scoring methods and compared them with a method I developed that automatically determines the ratio of sentences to select. Data selection is crucial for MT systems that aim to translate domain-specific texts. The data selection SMT models presented in this thesis were trained faster in comparison with training using full general domain data, had a smaller size, and performed on a par or better than the models trained using the full training data. | en |
dc.language.iso | en | de_DE |
dc.publisher | Staats- und Universitätsbibliothek Hamburg Carl von Ossietzky | de |
dc.rights | http://purl.org/coar/access_right/c_abf2 | de_DE |
dc.subject | Machine Translation | en |
dc.subject | Data Selection | en |
dc.subject | Domain Adaptation | en |
dc.subject.ddc | 004: Informatik | de_DE |
dc.title | Data Selection for Statistical Machine Translation | en |
dc.type | doctoralThesis | en |
dcterms.dateAccepted | 2021-12-01 | - |
dc.rights.cc | No license | de_DE |
dc.rights.rs | http://rightsstatements.org/vocab/InC/1.0/ | - |
dc.subject.gnd | Translation <Linguistik> | de_DE |
dc.type.casrai | Dissertation | - |
dc.type.dini | doctoralThesis | - |
dc.type.driver | doctoralThesis | - |
dc.type.status | info:eu-repo/semantics/publishedVersion | de_DE |
dc.type.thesis | doctoralThesis | de_DE |
tuhh.type.opus | Dissertation | - |
thesis.grantor.department | Informatik | de_DE |
thesis.grantor.place | Hamburg | - |
thesis.grantor.universityOrInstitution | Universität Hamburg | de_DE |
dcterms.DCMIType | Text | - |
dc.identifier.urn | urn:nbn:de:gbv:18-ediss-101970 | - |
datacite.relation.IsDerivedFrom | https://www.statmt.org/wmt14/medical-task/ | de_DE |
datacite.relation.IsDerivedFrom | https://www.statmt.org/wmt17/biomedical-translation-task.html | de_DE |
datacite.relation.IsDerivedFrom | https://www.statmt.org/wmt16/biomedical-translation-task.html | de_DE |
datacite.relation.IsDerivedFrom | https://www.statmt.org/wmt18/biomedical-translation-task.html | de_DE |
datacite.relation.IsDerivedFrom | https://www.statmt.org/wmt16/it-translation-task.html | de_DE |
datacite.relation.IsDerivedFrom | https://opus.nlpl.eu/index.php | de_DE |
datacite.relation.IsDerivedFrom | https://www.statmt.org/wmt13/translation-task.html | de_DE |
datacite.relation.IsDerivedFrom | https://ufal.mff.cuni.cz/ufal_medical_corpus | de_DE |
datacite.relation.IsDerivedFrom | https://www.statmt.org/wmt19/biomedical-translation-task.html | de_DE |
datacite.relation.IsDerivedFrom | https://github.com/jhlau/doc2vec | de_DE |
datacite.relation.IsDerivedFrom | http://ixa2.si.ehu.eus/stswiki/index.php/STSbenchmark | de_DE |
datacite.relation.IsDerivedFrom | https://alt.qcri.org/semeval2017/task1/index.php?id=data-and-tools | de_DE |
item.fulltext | With Fulltext | - |
item.creatorOrcid | Duma, Mirela-Stefania | - |
item.advisorGND | Menzel, Wolfgang | - |
item.advisorGND | Vertan, Cristina | - |
item.advisorGND | von Hahn, Walther | - |
item.languageiso639-1 | other | - |
item.creatorGND | Duma, Mirela-Stefania | - |
item.grantfulltext | open | - |
Enthalten in den Sammlungen: | Elektronische Dissertationen und Habilitationen |
Dateien zu dieser Ressource:
Datei | Beschreibung | Prüfsumme | Größe | Format | |
---|---|---|---|---|---|
Dissertation_SD.pdf | d8161f69b7f99455391477cb4b23072a | 2.66 MB | Adobe PDF | Öffnen/Anzeigen |
Diese Publikation steht in elektronischer Form im Internet bereit und kann gelesen werden. Über den freien Zugang hinaus wurden durch die Urheberin / den Urheber keine weiteren Rechte eingeräumt. Nutzungshandlungen (wie zum Beispiel der Download, das Bearbeiten, das Weiterverbreiten) sind daher nur im Rahmen der gesetzlichen Erlaubnisse des Urheberrechtsgesetzes (UrhG) erlaubt. Dies gilt für die Publikation sowie für ihre einzelnen Bestandteile, soweit nichts Anderes ausgewiesen ist.
Info
Seitenansichten
4.435
Letzte Woche
Letzten Monat
geprüft am 02.07.2025
Download(s)
329
Letzte Woche
Letzten Monat
geprüft am 02.07.2025
Werkzeuge