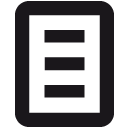
DC Element | Wert | Sprache |
---|---|---|
dc.contributor.advisor | Köhl, Michael (Prof. Dr.) | |
dc.contributor.author | Di Lallo, Giulio | |
dc.date.accessioned | 2020-10-19T13:20:06Z | - |
dc.date.available | 2020-10-19T13:20:06Z | - |
dc.date.issued | 2017 | |
dc.identifier.uri | https://ediss.sub.uni-hamburg.de/handle/ediss/7611 | - |
dc.description.abstract | Reducing Emissions from Deforestation and forest Degradation (REDD) is the major international political achievement to protect tropical forests’ carbon stocks. The basic idea of REDD+ is to pay forest owners (either through national government funds or directly) to reduce forest emissions and increase forest carbon sequestration. Such a simple idea is facing a number of challenges. This thesis analyses some of the forest monitoring technical challenges that countries can face, particularly during the early phases of REDD+ projects. The objective of this thesis is to provide a better understanding on approaches that could enable effective planning and implementation of monitoring activities. In doing so, this cumulative thesis focuses on three main concepts related to monitoring, reporting, and verification (MRV) systems: innovation, inclusion, and efficiency. Scientific and technological innovations support MRV systems, providing effective instruments to design and execute REDD+. Inclusion refers to the possibility of tropical countries or provinces to participate in REDD+; in fact, the capacity to implement a reliable MRV system determines the possibility of joining and executing an effective REDD+ program. Finally, pursuing efficiency is paramount, since most developing countries grapple with a shortage of resources, and the REDD+ mechanism copes even with a critical lack of finance. The first article presents a model, which using only available and easily accessible data and software, predicts the risk of deforestation. To predict the risk of deforestation, the model uses ten independent variables (called predictors), extracted from remotely sensed data. Environmental, social, demographic, and economic variables were incorporated in the model and used as proxies of deforestation. The model combines a machine learning approach and GIS. The machine learning approach is random forests; it is a decision tree–based method, which combining many classification trees produces a prediction of the variable of interest. We adopted random forests due to the strong non-linear relationships between the variables and because it supports evidence-based, data-driven decisions and is therefore often used in decision-making processes. We tested the model using data from Nicaragua. Results show that the accuracy of the model in predicting areas under moderate and high risk of deforestation can be considered satisfactory for some REDD+ purposes, e.g., when identifying potential target areas for REDD+ projects. Furthermore, the adoption of the model may be effective in the first phase of projects: when a country is still developing the capacity to build its own sound and accurate datasets. Therefore, the model is suitable for a stepwise implementation approach of REDD+ projects in regions with limited availability of data, capital, technical infrastructure, or human capacities. Stepwise approaches are needed to overcome existing data and capacity gaps and enable a wider participation to REDD+. Adopting an innovative model can improve efficiency and promote inclusion by exploiting already available data, by applying powerful methods to handle data, and by using open source software. The second article examines three key factors affecting the generation of forest carbon credits from REDD+. The factors are (i) setting Reference Levels (RLs); (ii) supplying of emission reduction due to REDD+; (iii) uncertainties in forest carbon emissions estimates. This article includes two analyses: a simulation study and a sensitivity analysis . In the simulation study, the interrelationships between the costs of forest carbon monitoring, the associated reliability, and the resulting accountable carbon credits were investigated. We assumed the employment of both Lidar data and passive optical data. Findings of the simulation study highlight that combining statistically rigorous sampling methods with Lidar data can significantly boost the accountable amount of forest carbon credits that can be claimed. In fact, the generation of carbon credits is mainly affected by the uncertainties of the estimate of forest area and carbon stock changes per unit of area. We found that innovative monitoring techniques have a positive effect on the efficiency of MRV systems, and that despite having a larger initial cost, the investment in MRV system, based on Lidar, could be paid-off by the potential result-based payments. Conceiving an MRV system as an investment can encourage the implementation of well-defined, long-term monitoring strategies. In the sensitivity analysis, the above-mentioned three factors are ranked according to their impact on the generation of carbon credits. Findings show that the amounts of avoided emissions under a REDD+ scheme mainly vary according to the monitoring technique adopted; nevertheless, RLs have a nearly equal influence. The target for reduction of emissions showed a relatively minor impact on the generation of carbon credits, particularly when coupled with low RLs. | en |
dc.language.iso | en | en |
dc.publisher | Staats- und Universitätsbibliothek Hamburg Carl von Ossietzky | |
dc.rights | http://purl.org/coar/access_right/c_abf2 | |
dc.subject | REDD+ | en |
dc.subject | remote sensing | en |
dc.subject | deforestation | en |
dc.subject | random forests | en |
dc.subject | land-use modeling | en |
dc.subject.ddc | 333.7 Natürliche Ressourcen, Energie und Umwelt | |
dc.title | Efficient, innovative, and inclusive options to overcome monitoring challenges in the early phases of REDD+ | en |
dc.title.alternative | Effiziente, innovative und umfassende Möglichkeiten zur Überwindung von Herausforderungen in frühen Erhebungsphasen von REDD+ | de |
dc.type | doctoralThesis | |
dcterms.dateAccepted | 2018-03-02 | |
dc.rights.cc | No license | |
dc.rights.rs | http://rightsstatements.org/vocab/InC/1.0/ | |
dc.subject.bcl | 48.00 Land- und Forstwirtschaft: Allgemeines | |
dc.type.casrai | Dissertation | - |
dc.type.dini | doctoralThesis | - |
dc.type.driver | doctoralThesis | - |
dc.type.status | info:eu-repo/semantics/publishedVersion | |
dc.type.thesis | doctoralThesis | |
tuhh.opus.id | 9049 | |
tuhh.opus.datecreation | 2018-04-03 | |
tuhh.type.opus | Dissertation | - |
thesis.grantor.department | Biologie | |
thesis.grantor.place | Hamburg | |
thesis.grantor.universityOrInstitution | Universität Hamburg | |
dcterms.DCMIType | Text | - |
tuhh.gvk.ppn | 1027763316 | |
dc.identifier.urn | urn:nbn:de:gbv:18-90490 | |
item.creatorOrcid | Di Lallo, Giulio | - |
item.creatorGND | Di Lallo, Giulio | - |
item.languageiso639-1 | other | - |
item.fulltext | With Fulltext | - |
item.advisorGND | Köhl, Michael (Prof. Dr.) | - |
item.grantfulltext | open | - |
Enthalten in den Sammlungen: | Elektronische Dissertationen und Habilitationen |
Dateien zu dieser Ressource:
Datei | Beschreibung | Prüfsumme | Größe | Format | |
---|---|---|---|---|---|
Dissertation.pdf | 4813245a1c9d394ab973201d16372a49 | 2.46 MB | Adobe PDF | Öffnen/Anzeigen |
Diese Publikation steht in elektronischer Form im Internet bereit und kann gelesen werden. Über den freien Zugang hinaus wurden durch die Urheberin / den Urheber keine weiteren Rechte eingeräumt. Nutzungshandlungen (wie zum Beispiel der Download, das Bearbeiten, das Weiterverbreiten) sind daher nur im Rahmen der gesetzlichen Erlaubnisse des Urheberrechtsgesetzes (UrhG) erlaubt. Dies gilt für die Publikation sowie für ihre einzelnen Bestandteile, soweit nichts Anderes ausgewiesen ist.
Info
Seitenansichten
331
Letzte Woche
Letzten Monat
geprüft am 03.04.2025
Download(s)
137
Letzte Woche
Letzten Monat
geprüft am 03.04.2025
Werkzeuge