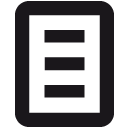
Titel: | Protein Structure Prediction using Coarse Grain Force Fields | Sonstige Titel: | Protein Strukturvorhersage mit grobkörnigen Kraftfeldern | Sprache: | Englisch | Autor*in: | Mahmood, Nasir | Erscheinungsdatum: | 2009 | Tag der mündlichen Prüfung: | 2010-02-12 | Zusammenfassung: | Protein structure prediction is one of the classic problems from computational chemistry. Experimental methods are the most accurate in protein structure determination but they are expensive and slow. That makes computational methods (i.e. comparative modeling and ab initio or de novo modeling) significant. In ab initio methods, one tries to build three- dimensional protein models from scratch rather than modeling them onto known structures. There are two aspects to this problem: 1) the score or quasi-energy function and 2) the search method. Our interest has been the development of quasi-energy functions. These could be seen as low-resolution special purpose coarse-grain or mesoscopic force fields, but they are rather different to most approaches. There is no strict physical model and no assumption of Boltzmann statistics. Instead, there is a mixture of Bayesian probabilities based on normal and discrete distributions. This has an interesting consequence. If one works with a method such as Monte Carlo, one can base the acceptance criterion directly on the ratio of calculated probabilities. Under a Bayesian framework, the probabilistic descriptions of the most probable set of classes were found by the classification of 1.5 million protein fragments, each fragment consists of 7 residues at maximum. These fragments were extracted from the known protein structures (with sequence identity less than 50% to each other) in the Protein Data Bank (PDB). Sequence, structure (phi and psi dihedral angles of the backbone) and solvation features of the fragments were modeled by multi-way discrete, bivariate normal, and simple normal distributions, respectively. An expectation minimization (EM) algorithm was used to find the most probable set of parameters for a given set of classes and the most probable set of classes in the fragment data irrespective of parameters. With the obtained classification, one can calculated the probability of a protein conformation as a product of the sums of probabilities of its constituent fragments across all classes. The ratio of these probabilities then allows us to replace the ratio which is derived from the Boltzmann statistics in traditional Metropolis Monte Carlo methods. The search method, simulated annealing Monte Carlo, makes three kinds of moves (i.e. biased, unbiased, and ’controlled’) to explore the conformational space. It has an artificial scheme to control the smoothness of the distributions. In initial results, the score function with sequence and structure terms only could produce protein-like models of the target sequences. Interestingly, these rather less compact models had good predictions of secondary structures. Incorporation of solvation term into the score function led to the generation of comparatively compact and sometimes native-like models, particularly for small targets. Models for relatively large and hard targets could also be generated with close secondary structure predictions. Secondary structures, particularly beta sheets, in these models often failed to properly pack themselves in the overall globular conformations. An ad hoc hydrogen bonding term based on an electrostatic model was introduced to entertain the long-range interactions. It could not make much difference probably due to its inconsistency with the score function. |
URL: | https://ediss.sub.uni-hamburg.de/handle/ediss/3002 | URN: | urn:nbn:de:gbv:18-46140 | Dokumenttyp: | Dissertation | Betreuer*in: | Torda, Andrew (Prof. Dr.) |
Enthalten in den Sammlungen: | Elektronische Dissertationen und Habilitationen |
Dateien zu dieser Ressource:
Datei | Beschreibung | Prüfsumme | Größe | Format | |
---|---|---|---|---|---|
NasirMahmood_Dissertation.pdf | 3fd31062d3257fee5168452a3130308b | 4.11 MB | Adobe PDF | Öffnen/Anzeigen |
Diese Publikation steht in elektronischer Form im Internet bereit und kann gelesen werden. Über den freien Zugang hinaus wurden durch die Urheberin / den Urheber keine weiteren Rechte eingeräumt. Nutzungshandlungen (wie zum Beispiel der Download, das Bearbeiten, das Weiterverbreiten) sind daher nur im Rahmen der gesetzlichen Erlaubnisse des Urheberrechtsgesetzes (UrhG) erlaubt. Dies gilt für die Publikation sowie für ihre einzelnen Bestandteile, soweit nichts Anderes ausgewiesen ist.
Info
Seitenansichten
561
Letzte Woche
Letzten Monat
geprüft am 27.05.2025
Download(s)
94
Letzte Woche
Letzten Monat
geprüft am 27.05.2025
Werkzeuge